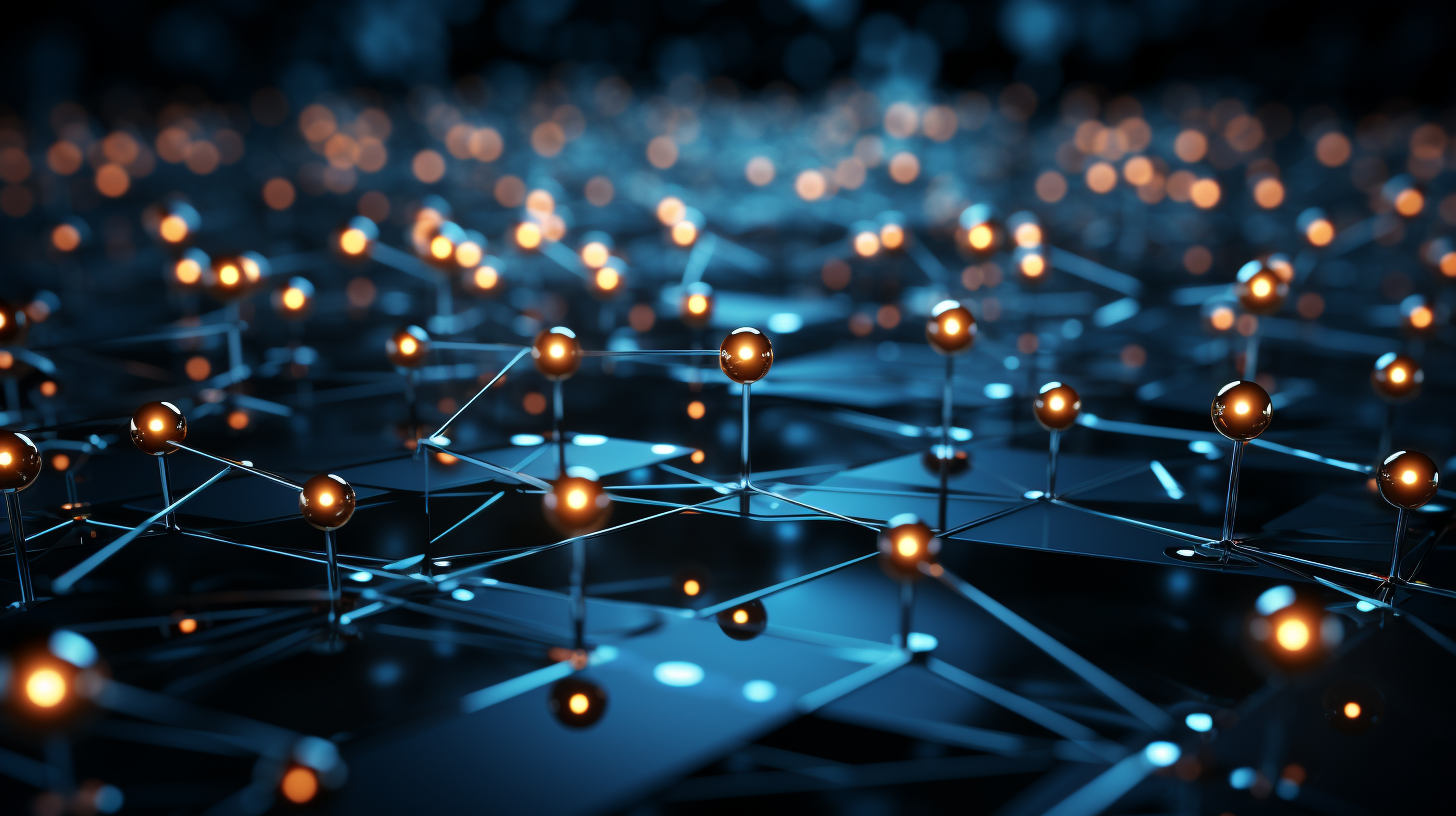
THE BLOG
Three Ways to Incorporate Your Own Data When Working with Generative AI
Midjourney / Antony Slumbers
A quick guide for business people.
Generative AI, via the likes of Open AI's GPT-4, Google's Gemini and Anthropic's Claude, is revolutionising the way businesses interact with and utilise artificial intelligence. These models can generate human-like text, assist with various tasks, and provide intelligent responses to user queries. However, to truly harness the power of generative AI for your business, it will often be the case that you wish to incorporate your own data into the process. In this article, we'll explore three methods to integrate your company's knowledge with generative AI and discuss the pros and cons of each approach.
Method 1: Uploading Documents within the Context of a Question
The simplest way to incorporate your own data when working with generative AI is to upload relevant documents within the context of a question. This approach involves providing the AI with specific excerpts or documents that contain the information needed to answer a given query. By doing so, you can ensure that the AI has access to the most relevant and up-to-date information from your company's knowledge base.
Pros:
Easy to implement and doesn't require any additional technical setup
Allows for targeted information sharing based on the specific question
Provides a straightforward way to control the information the AI uses to generate responses
Cons:
Limited by the size of the AI's context window, which may not accommodate larger documents or extensive knowledge bases
Requires manual effort to select and upload relevant documents for each question
The AI may still generate hallucinations if the provided context is incomplete or if it makes assumptions beyond the given information
Method 2: Connecting a ChatGPT Model to an Internal Directory of Documents
Another approach is to connect a model to an internal directory of documents. This method involves setting up a system where the AI can access and retrieve information from a centralised repository of your company's documents, such as reports, presentations, and knowledge base articles. One might do this via creating a 'GPT' via ChatGPT, or by interacting with a models API.
Pros:
Enables the AI to access a larger volume of information compared to uploading documents within the context of a question
Allows for a more automated and scalable way of incorporating your company's knowledge into the AI's responses
Provides a centralised and organised way to manage and update your company's knowledge base
Cons:
Requires technical setup and maintenance to connect the AI model to the document directory and ensure smooth information retrieval
The relevance of the retrieved information may be limited by traditional keyword-based search techniques
The AI may generate hallucinations if the retrieved documents are not entirely relevant or if there are gaps in the information
Method 3: Using a Vector Database and Retrieval-Augmented Generation (RAG) with Claude or A N Other model.
The most advanced and effective way to incorporate your own data when working with generative AI is to use a vector database and Retrieval-Augmented Generation (RAG). This approach involves converting your company's knowledge into high-dimensional vectors and storing them in a vector database. The RAG technique is then used to retrieve the most relevant information from the vector database and integrate it seamlessly with the AI's generation process.
Pros:
Offers the most accurate and relevant information retrieval based on semantic similarity
Enables a tight integration between the retrieved information and the AI's generation process, minimising the risk of hallucinations
Provides the highest level of customisation and fine-tuning to suit your specific business needs
Cons:
Requires significant technical expertise and resources to implement and maintain
Involves additional costs for setting up and running a vector database
Requires careful data preparation, preprocessing, and vectorisation to ensure optimal performance
Choosing the Right Approach
The best approach for incorporating your own data when working with generative AI depends on your business requirements, available resources, and the desired level of customisation and accuracy. If you prioritise ease of implementation and have a limited amount of data to incorporate, uploading documents within the context of a question may be sufficient. If you have a larger knowledge base and want a more automated solution, connecting a ChatGPT model to an internal directory of documents could be a good choice.
However, if your primary goal is to minimise hallucinations, maximise the relevance of the AI's responses, and have the highest level of customisation, using a vector database and RAG with one of the foundational models (GPT, Claude et al) is the most suitable option. This approach ensures that the AI is always grounded in your company's factual information and provides the most accurate and reliable responses.
Regardless of the approach you choose, it's essential to regularly review and update your company's knowledge base, monitor the AI's performance, and have human oversight to identify and correct any potential inaccuracies or hallucinations.
By incorporating your own data into generative AI, you can unlock the full potential of this technology and create AI-powered solutions that are tailored to your business needs.
—————————————-
A Note on Hallucinations:
When it comes to minimising hallucinations and ensuring accurate information (such as with a customer service chatbot), the choice of approach can have a significant impact.
A) Uploading documents within the context of a question:
Hallucination risk: Moderate to High
Explanation: While providing relevant documents within the context can help ground the responses in factual information, there is still a risk of hallucinations if the model makes assumptions or extrapolates beyond the provided context. The limited size of the context window may also lead to incomplete information, increasing the chances of hallucinations.
B) Connecting a ChatGPT model to an internal directory of documents:
Hallucination risk: Moderate
Explanation: By connecting the model to a directory of documents, you can provide a larger volume of information for the model to draw from. This can help reduce hallucinations compared to the first approach. However, the effectiveness of this approach depends on the quality and relevance of the documents retrieved by the keyword-based search. If the retrieved documents are not entirely relevant or if there are gaps in the information, the model may still generate hallucinations.
C) Using a vector database and RAG with Claude:
Hallucination risk: Low
Explanation: The combination of a vector database and RAG offers the best chance of minimising hallucinations. The vector database enables more accurate and relevant information retrieval based on semantic similarity, reducing the chances of missing important context. The RAG technique ensures that the retrieved information is tightly integrated with the generation process, keeping the model grounded in the factual information stored in the vector database. This approach allows for more control over the information the model uses to generate responses, minimising the risk of hallucinations.
Why Real Estate Needs a New Mindset to Succeed with Generative AI
Midjourney / Antony Slumbers
STOP!!!
I need you to take a deep breath and work through this step by step.
Those of you who, like me, spend too long talking to large language models, will recognise this counterintuitive phrase.
An instruction to an inanimate object to do something we know it cannot, but that weirdly, does produce superior outcomes.
What is going on? What delusion is being played out here? It’s a machine yet telling it to take a deep breath makes it work better.
What’s going on is that you ARE dealing with software, but not software that behaves as you have come to expect.
And that’s why, most likely, you are making mistakes in your plans to incorporate it into your business.
Historically software has been very deterministic. It does A, then B, then C. And it does the same thing over and over again. So we can easily decide what we want it to do and then expect it to do just that.
But large language models DO NOT behave like this. You don’t know if they will do A, then B, then C and you certainly do not know if they will do the same thing twice.
You also don’t know if they are going to tell you the truth. Ask the wrong sort of question, in the wrong sort of way, and they’ll give you an answer, a very plausible one. But it will have little to do with the truth.
Conversely, they will also make things up when you WANT them to - ‘give me 10 ideas about XYZ’.
So is that 'hallucination' bug actually a feature?
Can you have creativity without imagining things?
Either way, unless you have deeply internalised the actualité of what you are dealing with, you are going to come a cropper.
And you cannot deeply internalise the actualité of large language models unless you have used them extensively yourself, in a myriad of ways.
Which brings us back to STOP!!!
I am hearing and reading many accounts of real estate companies saying they are building this or that application, but doing so in a manner that suggests only a tendentious familiarity with the above. Not least of all a general lack of awareness as to what is generative and what is not generative AI (Generative AI is a creative tool, whereas Predictive AI is an analytical tool. That's quite some difference)
Which is of course understandable. Everyone is forever under pressure to deliver. And when we’re talking ‘flavour of the month’ the pressure can be intense.
But the outcomes will not be good. We need to approach generative AI from a different direction.
And that involves two things; practice and patience.
Practice in that you need to have as many people as possible within your company trying to incorporate using a language model in everything they do. You need to see what emerges from this. What works, what does not, what is surprising, what is disappointing. Where ‘might’ these things REALLY be useful? What should we get them to do, what should we do together, and what should we keep for ourselves?Where will they allow us to do something faster, better, cheaper? And much more importantly, where might they enable us to do something we could not do before? And what might that mean?
And patience in that Claude 3 has just been released but soon (in weeks maybe months, but not a year) we will have ChatGPT 5 and Gemini 2. And we’ve already been told, by Sam Altman himself, not to try and make certain hard and complicated things work today that the next version, coming soon, will happen right out of the box.
Bloomberg spend a fortune on BloombergGPT, their own customised version of GPT 3.5, only to find that today’s GPT 4 Turbo beats it for $20 a month.
So practice and be patient.
This is the fastest developing technology in history but it is more like an alien being than anything else we are familiar with. There is no user guide because the developers do not know what it can, or cannot, do. It is an emerging technology.
Ultimately, use of these tools will be embedded in everything your company does, and everyone in your company will use them in everything they do. But for now, the best advice is to use them, learn about them and see what comes from that. Only then should you be trying to productise them.
So ….. take a deep breath and let’s work through this step by step.
Why Real Estate Needs a New Mindset to Succeed with Generative AI
Midjourney / Antony Slumbers
STOP!!!
I need you to take a deep breath and work through this step by step.
Those of you who, like me, spend too long talking to large language models, will recognise this counterintuitive phrase.
An instruction to an inanimate object to do something we know it cannot, but that weirdly, does produce superior outcomes.
What is going on? What delusion is being played out here? It’s a machine yet telling it to take a deep breath makes it work better.
What’s going on is that you ARE dealing with software, but not software that behaves as you have come to expect.
And that’s why, most likely, you are making mistakes in your plans to incorporate it into your business.
Historically software has been very deterministic. It does A, then B, then C. And it does the same thing over and over again. So we can easily decide what we want it to do and then expect it to do just that.
But large language models DO NOT behave like this. You don’t know if they will do A, then B, then C and you certainly do not know if they will do the same thing twice.
You also don’t know if they are going to tell you the truth. Ask the wrong sort of question, in the wrong sort of way, and they’ll give you an answer, a very plausible one. But it will have little to do with the truth.
Conversely, they will also make things up when you WANT them to - ‘give me 10 ideas about XYZ’.
So is that 'hallucination' bug actually a feature?
Can you have creativity without imagining things?
Either way, unless you have deeply internalised the actualité of what you are dealing with, you are going to come a cropper.
And you cannot deeply internalise the actualité of large language models unless you have used them extensively yourself, in a myriad of ways.
Which brings us back to STOP!!!
I am hearing and reading many accounts of real estate companies saying they are building this or that application, but doing so in a manner that suggests only a tendentious familiarity with the above. Not least of all a general lack of awareness as to what is generative and what is not generative AI (Generative AI is a creative tool, whereas Predictive AI is an analytical tool. That's quite some difference)
Which is of course understandable. Everyone is forever under pressure to deliver. And when we’re talking ‘flavour of the month’ the pressure can be intense.
But the outcomes will not be good. We need to approach generative AI from a different direction.
And that involves two things; practice and patience.
Practice in that you need to have as many people as possible within your company trying to incorporate using a language model in everything they do. You need to see what emerges from this. What works, what does not, what is surprising, what is disappointing. Where ‘might’ these things REALLY be useful? What should we get them to do, what should we do together, and what should we keep for ourselves?Where will they allow us to do something faster, better, cheaper? And much more importantly, where might they enable us to do something we could not do before? And what might that mean?
And patience in that Claude 3 has just been released but soon (in weeks maybe months, but not a year) we will have ChatGPT 5 and Gemini 2. And we’ve already been told, by Sam Altman himself, not to try and make certain hard and complicated things work today that the next version, coming soon, will happen right out of the box.
Bloomberg spend a fortune on BloombergGPT, their own customised version of GPT 3.5, only to find that today’s GPT 4 Turbo beats it for $20 a month.
So practice and be patient.
This is the fastest developing technology in history but it is more like an alien being than anything else we are familiar with. There is no user guide because the developers do not know what it can, or cannot, do. It is an emerging technology.
Ultimately, use of these tools will be embedded in everything your company does, and everyone in your company will use them in everything they do. But for now, the best advice is to use them, learn about them and see what comes from that. Only then should you be trying to productise them.
So ….. take a deep breath and let’s work through this step by step.
5 Questions about AI and the Future of Architecture
Midjourney / Antony Slumbers
I was recently asked these five questions about the future of AI and the Architecture profession:
1.How do you envision AI impacting the architectural design process?
2.What are the most exciting benefits you see AI bringing to architectural design and construction?
3.What do you consider the biggest challenges architects will face when implementing AI technologies in their practices?
4.How can we achieve a balance between human creativity and intuition with AI-driven design solutions?
5.How do you see the role of architects changing in a future where AI plays a more prominent role?
Here are my answers:
1. How do you envision AI impacting the architectural design process?
AI will fundamentally transform the architectural design process by augmenting human creativity and enabling more efficient, optimised and responsive building design. Generative AI in particular will allow architects to rapidly explore a vast design space, generating countless design options based on specified parameters, constraints and goals around human-centricity, sustainability, adaptability, etc.
This will shift the architect's role from manually drafting designs to defining the right prompts, curating the generated options, and applying their human judgment, intuition and creativity to select and refine the best solutions. AI will be a powerful creative partner for architects.
2. What are the most exciting benefits you see AI bringing to architectural design and construction?
Some of the most exciting benefits I see AI bringing to architecture include:
Hyper-personalisation and human-centric optimisation of spaces.
By analysing granular data on environmental conditions, space utilisation, and individual occupant preferences, generative AI can create bespoke environments tailored to maximise user comfort, wellbeing and productivity. Spaces could dynamically adapt to changing needs.
Improved building performance and sustainability.
AI can help optimise energy efficiency, daylighting, natural ventilation and resource consumption by generating and simulating design options and control strategies. It can help achieve ambitious sustainability targets.
Expanded design exploration and innovation.
By rapidly generating and evaluating large numbers of design permutations, AI can help architects discover novel, high-performing solutions they may never have conceived of manually. It augments creativity.
Streamlined, accelerated workflows.
AI can automate tedious tasks, conduct rapid analyses and generate rich visualisations, freeing architects to focus on higher-level creative and strategic work. It can compress project timelines.
Continuous learning and adaptation.
With AI, buildings can become living entities that learn from occupant behaviour and self-optimise over time. Architecture can transcend static solutions to be dynamic and evolutionary.
3. What do you consider the biggest challenges architects will face when implementing AI technologies in their practices?
I see significant challenges architects must grapple with as they integrate AI:
Defining the right goals and guardrails.
Architects must frame the right optimisation objectives and constraints for AI to align with human values and priorities. Without clear ethical boundaries, we risk unintended negative consequences. Careful, values-based goal-setting is paramount.
Maintaining human agency and accountability.
As AI generates more of the design, architects must be vigilant to maintain authorship and creative control. Clear protocols will be needed for how to apply human oversight and when to override AI. Architects cannot abdicate responsibility for the end product.
Explicating and encoding design knowledge.
To leverage AI, architects must find ways to represent their domain expertise and design processes in machine-readable formats. This is not trivial and will require close collaboration with AI experts to create fit-for-purpose models.
Managing cultural acceptance and adoption.
Many architects may resist a technology they fear could devalue or displace their creative contributions. Firms will need change management strategies to build trust in AI as an enhancing rather than replacing force.
Up-skilling and re-skilling.
Architects will need to develop new competencies in data science, computational design, AI prompt crafting, etc. This will require significant investment in training and restructuring of architectural education.
4. How can we achieve a balance between human creativity and intuition with AI-driven design solutions?
Achieving a harmonious balance between human and AI in the creative process is perhaps the overarching challenge. I believe the key is pursuing AI as an augmenting tool for human designers, not an autonomous agent. The goal should be "centaur" teams where humans and AI work together to tackle creative problems, not AI creating in isolation.
This means keeping the human firmly in the loop - using AI to enhance our creative capabilities but reserving key decisions on design direction and refinement for human judgment. Architects should focus on the uniquely human skills of imagination, conceptualisation, aesthetic sensibility, and symbolic reasoning, while leveraging AI for exploration, optimisation, evaluation and crafting of details.
Organisationally, this points to integrated teams where AI specialists work hand-in-hand with designers, collaboratively evolving the AI to serve the human-centric design intent. There must be ongoing "meta-design" of the AI system itself as a living design tool attuned to the architects' creative preferences and needs.
5. How do you see the role of architects changing in a future where AI plays a more prominent role?
I see the role of architects elevating to be more akin to a "curator of AI-generated possibilities" or a "creative director of AI." Rather than drafting designs detail by detail, architects will specialise in:
Developing the overall creative vision and design concepts
Defining the parameters and criteria to guide AI-generated options
Curating and selecting the most promising AI outputs
Refining and synthesising selected options into coherent designs
Overseeing the "training" and evolution of the AI based on project learnings
Developing and communicating the story and experience of the spaces to clients and users
Upholding the ethical, social and environmental integrity of the design
Orchestrating the dynamic interplay of AI and human stakeholders
In many ways, AI will enable architects to transcend being just form-givers and problem-solvers to become stewards of holistic human-centered experiences. By offloading technical complexity to AI, architects can re-focus on the most humanistic aspects of design - the art, philosophy, psychology and beauty of the built environment.
They will be free to grapple with the deeper questions of what kinds of spaces we want to create for human flourishing, and to shape the AI towards those poetic and purposeful ends. In an AI-mediated world, maintaining that human point of view - keeping people and not just performance as the ultimate touchstone - will be the architect's most vital role.
In summary, while AI will undoubtedly disrupt and transform architecture in many ways, I believe it also offers tremendous opportunity to amplify the human-centric impact of their work. By wedding the efficiency and inventiveness of AI with the empathy and ethics of human creativity, we can create an architecture more responsive and uplifting to the human spirit than ever before.
The future is bright for firms that learn to wield AI towards profoundly human ends.
So you want to be in PropTech?
How to Ideate a PropTech Business using Generative AI
PropTech VC PI Labs released a report last week about ‘AI for a Greener Built World’ titled ‘Sustainably Intelligent’.
In it they broke down environmental use cases along the real estate value chain. In 7 Categories.
So I thought ‘how could AI help someone develop a business aimed at one of more of these defined use cases?’
I started with Category 1: Site Supply and Acquisition.
This listed six tasks required:
Localised environmental data and analysis
Identification and monitoring of biodiversity
Biodiversity management and intervention
Robotic insecticide alternatives
Soil quality and health analysis
Microclimate simulations
So for each of these I enlisted the help of Claude 3 Opus.
First off I defined to it what I meant by Generative and Predictive AI.
Then I asked the following questions, for each item:
Might this benefit from Generative AI?
Might this benefit from Predictive AI?
In every case there was a use for Generative and Predictive AI (this is not surprising with a GPT - a General Purpose Technology - they are pervasive)
So, then I needed to know:
What ‘Actions’ could Generative AI take?
What ‘Actions’ could Predictive AI take?
And
What inputs (data) would be required so that these ‘Actions’ could be undertaken?
What Data Sources are these inputs likely to come from?
Then finally I asked:
What technology stack would we need?
What would be the right evaluation metrics for this item?
What integration requirements, with other systems, would we need?
Having done this I needed all this information formatted as a table and available to be downloaded.
I then took this into Google Slides, and cleaned it up a bit before ending up with the table below.
You’ve now got a strong set of data to start thinking about your proposed PropTech business.
You can see how Generative and Predictive AI can be woven together to provide analytics and presentation/communication layers. You can see what value there is to create, what data sources you would require to make this happen, and the technological skills your team would need access to.
From this you should be able to ascertain whether 1) it’s possible 2) You could make it happen and 3) Does it look like there’s enough value there for all the work involved?
Don’t like this category? Then work your way through the other 6.
Then you will have covered off environmental use cases for AI along the real estate value chain!
Is it a GO?